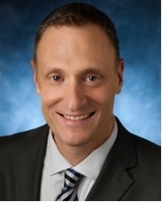
Bradley E. Aouizerat's additional information
-
-
BS, Microbiology/ Molecular Genetics - University of California at Los AngelesPhD, Microbiology/ Molecular Genetics/lmmunology - University of California at Los AngelesMAS, Master of Advance Science Research in Clinical - University of California at San Francisco
-
-
Oral-systemic health
-
-
American Heart AssociationAmerican Liver FoundationAmerican Pain SocietyAmerican Society for Human GeneticsInternational Association for the Study of Pain
-
-
Faculty Honors Awards
Excellence in Research Mentoring Faculty Teaching Award (2013)Excellence in Research Mentoring Faculty Teaching Award (Nominee) (2012)Excellence in Research Mentoring Faculty Teaching Award (Nominee) (2011)Most Dedicated Mentor Award, PMCTR Fellowship Program (2009)Early Career Investigator Award, Bayer Healthcare International (2006)Multidisciplinary Clinical Research Scholar, Roadmap K12 (2006)Early Career Faculty Award, Hellman Family (2005)Faculty Mentorship Award Nominee (2005)Young Investigator Award, National Hemophilia Foundation (2005)National Liver Scholar Award, American Liver Foundation (2004)Irvine H. Page Young Investigator Award (Finalist), American Heart Association (2004)Faculty Mentorship Award Nominee (2004)Sam and Rose Gilbert Fellowship, UCLA (1998)Warsaw Fellowship (1998) -
-
Publications
Altered Bacteria Abundance Is Associated With Outcomes in Head and Neck Squamous Cell Carcinoma
Failed retrieving data.Development of high-titer class-switched antibody responses to phosphorylated amino acids is prevalent in pancreatic ductal adenocarcinoma
Failed retrieving data.Differential Gene Expression in Response to AWARENESS: A Randomized Controlled Trial of an Intersectional Minority Stress Intervention
Failed retrieving data.Heterogeneous depressive symptom trajectories among women with type 2 diabetes: findings from the Women’s Interagency HIV Study
Failed retrieving data.The low-density lipoprotein receptor-related protein-1 (LRP1) in Schwann cells controls mitochondria homeostasis in peripheral nerves
Failed retrieving data.Neighborhood-Level Adversity and Inflammation Among Sexual Minority Men Living With HIV
Failed retrieving data.Perineural Invasion Exhibits Traits of Neurodegeneration
Failed retrieving data.Poor Sleep Quality is Associated with Frailty Among Women with and without HIV
Failed retrieving data.A simple phylogenetic approach to analyze hypermutated HIV proviruses reveals insights into their dynamics and persistence during antiretroviral therapy
Failed retrieving data.Artificial Intelligence Applications in Oral Cancer and Oral Dysplasia
Failed retrieving data.