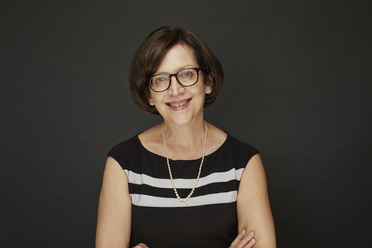
Janet H Van Cleave
MBA PhD
Assistant Professor
janet.vancleave@nyu.edu
1 212 992 7340
433 First Ave
New York, NY 10010
United States
Janet H Van Cleave's additional information
-
-
Janet Helen Van Cleave, PhD, is an assistant professor at NYU Rory Meyers College of Nursing. Her program of research is focused on symptom science and mHealth technology use in cancer. She is an oncology nurse and nurse scientist whose career goal is to improve the quality of care for patients with cancer.
Van Cleave developed the Electronic Patient Visit Assessment (ePVA)© for head and neck cancer for early detection and intervention for debilitating symptoms. Her program of research has received both federal and foundation funding. She has published in high-impact scientific journals and online magazines like WIRED.
Among her many awards, she received the Poster of Distinction by the International Federation of Head and Neck Oncologic Societies and the 2014 CANCER NURSING Research Award. She was a fellow of the American Psychosocial Oncology Society Conference in New Orleans, LA.
Van Cleave received her PhD from Yale University and completed post-doctoral training at the NewCourtland Center for Transitions and Health at the University of Pennsylvania School of Nursing. She earned her MS and BS in nursing from the University of Pennsylvania.
-
-
Post-Doctoral Research Fellow - University of Pennsylvania (2010)PhD - Yale University (2008)MSN - University of Pennsylvania (1995)BSN - University of Pennsylvania (Summa Cum Laude, 1994)Diploma of Nursing - St. Luke’s Hospital School of Nursing (1983)MBA - University of Kansas (1978)BA - Kansas State University (1976)
-
-
Gerontology
-
-
Academy HealthAmerican Psychosocial Oncology SocietyGerontological Society of AmericaInternational Association for the Study of PainOncology Nursing Society
-
-
Faculty Honors Awards
Mayday Pain & Society Fellowship, The Mayday Fund (2019)ENRS/Nursing Research Authorship Award, Eastern Nursing Research Society (2017)Poster of Distinction, International Federation of Head and Neck Oncologic Societies (2014)Fellowship, American Psychosocial Oncology Society Conference, New Orleans, LA (2010)Scholarship, 8th National Conference on Cancer Nursing Research, John A. Harford Foundation Policy Leadership Institute Oncology Nursing Society/American Cancer Society (2009)Outstanding Colleague, Mount Sinai Medical Center (2004)Best Article, Oncology Nursing Society Special Interest Group Newsletter Editor (2004)Nominee, Clinical Excellence Award, Mount Sinai Medical Center (2002)Unit Recognition Award for Special Clinics, Philadelphia Veterans Affairs Medical Center (2000)Health Professional Scholarship, Department of Veterans Affairs (1994)Sigma Theta Tau, University of Pennsylvania School of Nursing (1994)Joan Ethel Huebner Award for High GPA, University of Pennsylvania School of Nursing (1994) -
-
Publications
The association between the mental health disorders, substance abuse, and tobacco use with head & neck cancer stage at diagnosis
AbstractWoersching, J., Van Cleave, J. H., Gonsky, J. P., Ma, C., Haber, J., Chyun, D., & Egleston, B. L. (2025). Cancer Causes and Control, 36(3), 231-242. 10.1007/s10552-024-01921-0AbstractPurpose: Mental health disorders, substance abuse, and tobacco use are prevalent in the US population. However, the association between these conditions and head and neck cancer (HNC) stage is poorly understood. This research aims to uncover the relationship between pre-existing mental health disorders, substance abuse, and tobacco use and HNC stage at diagnosis in patients receiving care in an integrated, public safety-net healthcare system. Methods: This study was a secondary data analysis of linked hospital tumor registries and electronic health record (EHR) data. The study’s primary independent variables were the comorbidities of mental health disorders, substance abuse, and tobacco use. The dependent variable was HNC stage at diagnosis, operationalized as early stage (i.e., stages I, II, and III) and advanced stage (stage IV, IVA, IVB, or IVC). The analysis included multivariable logistic regression adjusted for covariates of demographic variables, tumor anato Results: The study population consisted of 357 patients with median age of 59 years, and was primarily male (77%), diverse (Black or African American 41%; Hispanic 22%), and from neighborhoods with low income (median average annual household income $39,785). Patients with a history of mental health disorders with or without tobacco use had significantly lower odds of advanced stage HNC at diagnosis (adjusted OR = 0.35, 95% Confidence Interval [CI]: 0.17–0.72.) while patients with a history of substance abuse with or without tobacco use had significantly higher odds of advanced stage HNC at diagnosis (adjusted OR 1.41, 95% CI: 1.01–1.98) than patients with no history of mental health disorders, substance abuse, or tobacco use. Conclusions: The relationship between HNC stage at diagnosis and the comorbidities of mental health disorders, substance abuse, or tobacco differs depending on the type and co-occurrence of these comorbidities. These findings demonstrate the need for innovative care delivery models and education initiatives tailored to meet the needs of patients with mental health disorders, substance abuse, and tobacco use that facilitate early detection of HNC.Data Quality of Automated Comorbidity Lists in Patients With Mental Health and Substance Use Disorders
AbstractWoersching, J., Van Cleave, J. H., Egleston, B., Ma, C., Haber, J., & Chyun, D. (2022). CIN - Computers Informatics Nursing, 40(7), 497-505. 10.1097/CIN.0000000000000889AbstractEHRs provide an opportunity to conduct research on underrepresented oncology populations with mental health and substance use disorders. However, a lack of data quality may introduce unintended bias into EHR data. The objective of this article is describe our analysis of data quality within automated comorbidity lists commonly found in EHRs. Investigators conducted a retrospective chart review of 395 oncology patients from a safety-net integrated healthcare system. Statistical analysis included κ coefficients and a condition logistic regression. Subjects were racially and ethnically diverse and predominantly used Medicaid insurance. Weak κ coefficients (κ = 0.2-0.39, P <.01) were noted for drug and alcohol use disorders indicating deficiencies in comorbidity documentation within the automated comorbidity list. Further, conditional logistic regression analyses revealed deficiencies in comorbidity documentation in patients with drug use disorders (odds ratio, 11.03; 95% confidence interval, 2.71-44.9; P =.01) and psychoses (odds ratio, 0.04; confidence interval, 0.02-0.10; P <.01). Findings suggest deficiencies in automatic comorbidity lists as compared with a review of provider narrative notes when identifying comorbidities. As healthcare systems increasingly use EHR data in clinical studies and decision making, the quality of healthcare delivery and clinical research may be affected by discrepancies in the documentation of comorbidities.Symptoms of Malignant Fungating Wounds and Functional Performance among Patients with Advanced Cancer: An Integrative Review from 2000 to 2019
AbstractTilley, C. P., Fu, M. R., Van Cleave, J., Crocilla, B. L., & Comfort, C. P. (2020). Journal of Palliative Medicine, 23(6), 848-862. 10.1089/jpm.2019.0617AbstractIntroduction: Malignant fungating wounds (MFWs), non-healing wounds caused by aggressive proliferation of malignant tumors, afflict 5%-14.5% of patients with advanced cancer. We conducted an integrative review to evaluate the level of evidence of peer-reviewed literature published from 2000 to 2019 on symptoms of MFWs, and the impact of the symptoms on functional performance among patients with advanced cancer. Methods: Four electronic databases were searched and 1506 articles were retrieved. A total of 1056 abstracts were screened for relevance and a full review of the 26 articles was performed. A total of 12 articles met inclusion criteria. An established quality assessment tool was used to rate the quality of the included studies. Results: The overall quality of the included 12 studies was adequate. This integrative review of the literature provided strong evidence that patients with MFWs suffered multiple symptoms, including pain, odor, exudate, bleeding, pruritus, perceived wound status, perceived bulk effect and lymphedema. Quantitative research was not able to capture the occurrence and characteristics of all the identified symptoms. There was a lack of quantitative research on the impact of MFWs and symptoms on patients' functional performance. Yet, qualitative studies provided vivid description of how the symptoms negatively affected patients' functional performance. Future research should develop a clinical tool that enables the comprehensive assessment of symptoms of MFWs. Well-designed quantitative research is needed to delineate the impact of symptoms of MFWs on patients' functional performance to ensure quality palliative care.The Experience of Being Aware of Disease Status in Women with Recurrent Ovarian Cancer: A Phenomenological Study
AbstractFinlayson, C. S., Fu, M. R., Squires, A., Applebaum, A., Van Cleave, J., O’Cearbhaill, R., & Derosa, A. P. (2019). Journal of Palliative Medicine, 22(4), 377-384. 10.1089/jpm.2018.0127AbstractBackground: Awareness of disease status has been identified as a factor in the treatment decision-making process. Women with recurrent ovarian cancer are facing the challenge of making treatment decisions throughout the disease trajectory. It is not understood how women with ovarian cancer perceive their disease and subsequently make treatment decisions. Purpose: The purpose of this phenomenological study was to understand the lived experience of women with recurrent ovarian cancer, how they understood their disease and made their treatment decisions. Methods: A qualitative design with a descriptive phenomenological method was used to conduct 2 in-depth interviews with 12 women (n = 24 interviews). Each interview was ∼60 minutes and was digitally recorded and professionally transcribed. Data collection focused on patients' understanding of their disease and how patients participated in treatment decisions. A modified version of Colaizzi's method of phenomenological reduction guided data analysis. Results: Three themes emerged to describe the phenomenon of being aware of disease status: (1) perceiving recurrent ovarian cancer as a chronic illness, (2) perceived inability to make treatment decisions, and (3) enduring emotional distress. Conclusions and Implications: This study revealed how 12 women conceptualized recurrent ovarian cancer as a chronic disease and their perceived inability to make treatment decisions because of lack of information and professional qualifications, resulting in enduring emotional distress. Future research should replicate the study to confirm the persistence of the themes for racially, ethnically, and religiously diverse patient samples and to improve understanding of awareness of disease status and decision-making processes of patients.Mental health and substance use disorders in patients diagnosed with cancer: An integrative review of healthcare utilization
AbstractWoersching, J., Van Cleave, J. H., Haber, J., & Chyun, D. (2019). Oncology Nursing Forum, 46(3), 365-383. 10.1188/19.ONF.365-383AbstractPROBLEM IDENTIFICATION: The impact of mental health disorders (MHDs) and substance use disorders (SUDs) on healthcare utilization (HCU) in patients with cancer is an understudied phenomenon. LITERATURE SEARCH: A literature search of studies published prior to January 2018 that examined HCU in patients with preexisting MHDs or SUDs diagnosed with cancer was conducted. DATA EVALUATION: The research team evaluated 22 studies for scientific rigor and examined significant trends in HCU, as well as types of the MHD, SUD, and cancer studied. SYNTHESIS: The heterogeneity of HCU outcome measures, MHD, SUD, sample sizes, and study settings contributed to inconsistent study findings. However, study trends indicated higher rates of HCU by patients with depression and lower rates of HCU by patients with schizophrenia. In addition, the concept of HCU measures is evolving, addressing not only volume of health services, but also quality and efficacy. IMPLICATIONS FOR RESEARCH: Oncology nurses are essential to improving HCU in patients with MHDs and SUDs because of their close connections with patients throughout the stages of cancer care. Additional prospective studies are needed to examine specific MHDs and different types of SUDs beyond alcohol use, improving cancer care and the effectiveness of HCU in this vulnerable population.Machine learning for detection of lymphedema among breast cancer survivors
AbstractFu, M., Wang, Y., LI, C., Qiu, Z., Axelrod, D., Guth, A. A., Scagliola, J., Conley, Y. P., Aouizerat, B., Qiu, J. M., Yu, G., Van Cleave, J., Haber, J., & Cheung, Y. K. (2018). MHealth, 4. 10.21037/mhealth.2018.04.02AbstractBackground: In the digital era when mHealth has emerged as an important venue for health care, the application of computer science, such as machine learning, has proven to be a powerful tool for health care in detecting or predicting various medical conditions by providing improved accuracy over conventional statistical or expert-based systems. Symptoms are often indicators for abnormal changes in body functioning due to illness or side effects from medical treatment. Real-time symptom report refers to the report of symptoms that patients are experiencing at the time of reporting. The use of machine learning integrating real-time patient-centered symptom report and real-time clinical analytics to develop real-time precision prediction may improve early detection of lymphedema and long term clinical decision support for breast cancer survivors who face lifelong risk of lymphedema. Lymphedema, which is associated with more than 20 distressing symptoms, is one of the most distressing and dreaded late adverse effects from breast cancer treatment. Currently there is no cure for lymphedema, but early detection can help patients to receive timely intervention to effectively manage lymphedema. Because lymphedema can occur immediately after cancer surgery or as late as 20 years after surgery, real-time detection of lymphedema using machine learning is paramount to achieve timely detection that can reduce the risk of lymphedema progression to chronic or severe stages. This study appraised the accuracy, sensitivity, and specificity to detect lymphedema status using machine learning algorithms based on real-time symptom report.Methods: A web-based study was conducted to collect patients' real-time report of symptoms using a mHealth system. Data regarding demographic and clinical information, lymphedema status, and symptom features were collected. A total of 355 patients from 45 states in the US completed the study. Statistical and machine learning procedures were performed for data analysis. The performance of five renowned classification algorithms of machine learning were compared: Decision Tree of C4.5, Decision Tree of C5.0, gradient boosting model (GBM), artificial neural network (ANN), and support vector machine (SVM). Each classification algorithm has certain user-definable hyper parameters. Five-fold cross validation was used to optimize these hyper parameters and to choose the parameters that led to the highest average cross validation accuracy.Results: Using machine leaning procedures comparing different algorithms is feasible. The ANN achieved the best performance for detecting lymphedema with accuracy of 93.75%, sensitivity of 95.65%, and specificity of 91.03%.Conclusions: A well-trained ANN classifier using real-time symptom report can provide highly accurate detection of lymphedema. Such detection accuracy is significantly higher than that achievable by current and often used clinical methods such as bio-impedance analysis. Use of a well-trained classification algorithm to detect lymphedema based on symptom features is a highly promising tool that may improve lymphedema outcomes.Policy Research Challenges in Comparing Care Models for Dual-Eligible Beneficiaries
AbstractVan Cleave, J. H., Egleston, B. L., Brosch, S., Wirth, E., Lawson, M., Sullivan-Marx, E. M., & Naylor, M. D. (2017). Policy, Politics, and Nursing Practice, 18(2), 72-83. 10.1177/1527154417721909AbstractProviding affordable, high-quality care for the 10 million persons who are dual-eligible beneficiaries of Medicare and Medicaid is an ongoing health-care policy challenge in the United States. However, the workforce and the care provided to dual-eligible beneficiaries are understudied. The purpose of this article is to provide a narrative of the challenges and lessons learned from an exploratory study in the use of clinical and administrative data to compare the workforce of two care models that deliver home- and community-based services to dual-eligible beneficiaries. The research challenges that the study team encountered were as follows: (a) comparing different care models, (b) standardizing data across care models, and (c) comparing patterns of health-care utilization. The methods used to meet these challenges included expert opinion to classify data and summative content analysis to compare and count data. Using descriptive statistics, a summary comparison of the two care models suggested that the coordinated care model workforce provided significantly greater hours of care per recipient than the integrated care model workforce. This likely represented the coordinated care model's focus on providing in-home services for one recipient, whereas the integrated care model focused on providing services in a day center with group activities. The lesson learned from this exploratory study is the need for standardized quality measures across home- and community-based services agencies to determine the workforce that best meets the needs of dual-eligible beneficiaries.Adherence to Antiestrogen Oral Endocrine Therapy Among Older Women With Breast Cancer
Van Cleave, J., Elstein, N., & Brody, A. A. (2015). Nursing Research, 64(2), E72-E72. -
-
Media