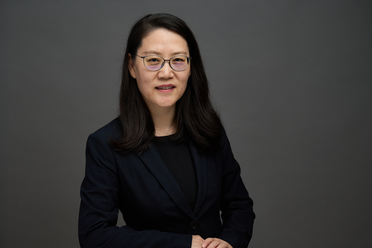
Yaguang Zheng
PhD RN
Assistant Professor
yaguang.zheng@nyu.edu
1 212 998 5170
433 FIRST AVENUE
NEW YORK, NY 10010
United States
Yaguang Zheng's additional information
-
-
Yaguang Zheng is an assistant professor at NYU Rory Meyers College of Nursing. Her research focuses on cardiometabolic risk reduction by leveraging mobile health, electronic health records, and data science techniques. Zheng has explored behavioral phenotypes through the use of wireless devices in clinical trials and real-world settings and their impacts on cardiometabolic disease prevention and management. Zheng’s initial work focused on lifestyle behavior changes through mobile health, more specifically, using mobile health for self-monitoring and its impact on weight-loss outcomes. After identifying a critical knowledge gap in the area of engagement with mobile health, Zheng conducted a pilot study that found that older adults were able to use multiple mobile devices to improve diabetes self-management, debunking traditional perceptions of older adults as being skeptical of multiple mobile technologies.
Zheng has also applied machine learning algorithms to analyze data from a large real-world sample that has yielded varied patterns of use of wireless devices over the course of a year, findings which are helping to target subgroups of individuals who need long-term engagement in using mobile health devices. More recently, Zheng has worked on electronic health record data, including mobile health data from wearable devices, like continuous glucose monitors, which has real-world application for clinical practice.
Prior to joining the NYU Rory Meyers faculty, Zheng was a postdoctoral scholar supported by NIH grant T32 NR008857 Technology: Research in Chronic and Critical Illness at the University of Pittsburgh School of Nursing.
Zheng earned her PhD at the University of Pittsburgh. She also received a Nursing Informatics Certificate during her postdoctoral training.
-
-
PhD, Nursing - University of Pittsburgh
-
-
ObesityDiabetesChronic diseaseInformatics
-
-
American Medical Informatics AssociationAmerican Heart AssociationAmerican Diabetes Association
-
-
Faculty Honors Awards
Post-doctoral trainee, Technology: Research in Chronic and Critical Illness (T32 NR008857) (2020)Ruth Perkins Kuehn Scholarship, Sigma Theta Tau, Eta Chapter (2014)New Investigator Travel Award, American Heart Association EPI/NPAM 2014 Scientific Sessions (2014) -
-
Publications
AI Applications for Chronic Condition Self-Management: Scoping Review
AbstractHwang, M., Zheng, Y., Cho, Y., & Jiang, Y. (2025). Journal of Medical Internet Research, 27, e59632. 10.2196/59632AbstractBACKGROUND: Artificial intelligence (AI) has potential in promoting and supporting self-management in patients with chronic conditions. However, the development and application of current AI technologies to meet patients' needs and improve their performance in chronic condition self-management tasks remain poorly understood. It is crucial to gather comprehensive information to guide the development and selection of effective AI solutions tailored for self-management in patients with chronic conditions.OBJECTIVE: This scoping review aimed to provide a comprehensive overview of AI applications for chronic condition self-management based on 3 essential self-management tasks, medical, behavioral, and emotional self-management, and to identify the current developmental stages and knowledge gaps of AI applications for chronic condition self-management.METHODS: A literature review was conducted for studies published in English between January 2011 and October 2024. In total, 4 databases, including PubMed, Web of Science, CINAHL, and PsycINFO, were searched using combined terms related to self-management and AI. The inclusion criteria included studies focused on the adult population with any type of chronic condition and AI technologies supporting self-management. This review was conducted following the PRISMA-ScR (Preferred Reporting Items for Systematic Reviews and Meta-Analyses Extension for Scoping Reviews) guidelines.RESULTS: Of the 1873 articles retrieved from the search, 66 (3.5%) were eligible and included in this review. The most studied chronic condition was diabetes (20/66, 30%). Regarding self-management tasks, most studies aimed to support medical (45/66, 68%) or behavioral self-management (27/66, 41%), and fewer studies focused on emotional self-management (14/66, 21%). Conversational AI (21/66, 32%) and multiple machine learning algorithms (16/66, 24%) were the most used AI technologies. However, most AI technologies remained in the algorithm development (25/66, 38%) or early feasibility testing stages (25/66, 38%).CONCLUSIONS: A variety of AI technologies have been developed and applied in chronic condition self-management, primarily for medication, symptoms, and lifestyle self-management. Fewer AI technologies were developed for emotional self-management tasks, and most AIs remained in the early developmental stages. More research is needed to generate evidence for integrating AI into chronic condition self-management to obtain optimal health outcomes.Association of Gum Treatment with Cognitive Decline and Dementia Risk among Older Adults with Periodontal Symptoms: A 12-Year Prospective Cohort Study
AbstractZhu, Z., Wang, K., Zheng, Y., Li, A., Wu, B., & Qi, X. (2025). Neuroepidemiology, 59(4), 1-10. 10.1159/000540086AbstractINTRODUCTION: This study examines the associations of gum treatment with cognitive decline and dementia risk among older adults with periodontal symptoms in the USA.METHODS: A cohort of 866 adults aged ≥50 with periodontal symptoms was recruited for the 2008 Health and Retirement Study "Dental Health Experimental Module" and followed until 2020. Cognitive function was assessed with the Telephone Interview for Cognitive Status (TICS). Dementia status was ascertained with the Langa-Weir algorithm based on TICS scores and proxy assessments. Linear mixed-effects model and multivariable Cox regression models were utilized to analyze the associations of gum treatment with cognitive decline and the risk of dementia, respectively.RESULTS: Of 866 participants (mean age 67.7, 61.4% women), 105 (12.1%) developed dementia with a median follow-up of 9 (IQR, 6-10) years. The dementia incidence rates were lower in the group with gum treatment (7.4 vs. 12.9 per 1,000 person-years). Compared with participants who did not have gum treatment, those with gum treatment experienced a decline in TICS score that was on average 0.025 (95% CI, 0.005-0.044) points less per year and a 38% lower incidence of dementia (hazard ratio, 0.62; 95% CI, 0.41-0.93). These associations were consistent across participants with a different severity of periodontal symptoms and sociodemographic characteristics (age, sex, race, ethnicity, and education) except for income levels.CONCLUSION: Prompt gum treatment for older adults with periodontal symptoms may be beneficial for their cognitive health.Classifying Continuous Glucose Monitoring Documents From Electronic Health Records
AbstractZheng, Y., Iturrate, E., Li, L., Wu, B., Small, W. R., Zweig, S., Fletcher, J., Chen, Z., & Johnson, S. B. (2025). Journal of Diabetes Science and Technology. 10.1177/19322968251324535AbstractBackground: Clinical use of continuous glucose monitoring (CGM) is increasing storage of CGM-related documents in electronic health records (EHR); however, the standardization of CGM storage is lacking. We aimed to evaluate the sensitivity and specificity of CGM Ambulatory Glucose Profile (AGP) classification criteria. Methods: We randomly chose 2244 (18.1%) documents from NYU Langone Health. Our document classification algorithm: (1) separated multiple-page documents into a single-page image; (2) rotated all pages into an upright orientation; (3) determined types of devices using optical character recognition; and (4) tested for the presence of particular keywords in the text. Two experts in using CGM for research and clinical practice conducted an independent manual review of 62 (2.8%) reports. We calculated sensitivity (correct classification of CGM AGP report) and specificity (correct classification of non-CGM report) by comparing the classification algorithm against manual review. Results: Among 2244 documents, 1040 (46.5%) were classified as CGM AGP reports (43.3% FreeStyle Libre and 56.7% Dexcom), 1170 (52.1%) non-CGM reports (eg, progress notes, CGM request forms, or physician letters), and 34 (1.5%) uncertain documents. The agreement for the evaluation of the documents between the two experts was 100% for sensitivity and 98.4% for specificity. When comparing the classification result between the algorithm and manual review, the sensitivity and specificity were 95.0% and 91.7%. Conclusion: Nearly half of CGM-related documents were AGP reports, which are useful for clinical practice and diabetes research; however, the remaining half are other clinical documents. Future work needs to standardize the storage of CGM-related documents in the EHR.Efficacy of a culturally tailored intervention on perceived stigma among women living with HIV/AIDS in China: A randomized clinical trial
AbstractYang, Z., Han, S., Qi, X., Wang, J., Xu, Z., Mao, W., Zheng, Y., Zhang, Y., Wu, B., & Hu, Y. (2025). Ethics in Science and Medicine, 374, 118072. 10.1016/j.socscimed.2025.118072AbstractBACKGROUND: Despite evidence supporting the efficacy of culturally tailored interventions in reducing stigma, such approaches are lacking for women living with HIV/AIDS (WLWHAs) in China. We conducted this study to determine the efficacy of the culturally tailored Helping Overcome Perceived Stigma (HOPES) intervention in reducing perceived stigma among WLWHAs in China.METHODS: A single-blinded, two-arm parallel-group randomized clinical trial was conducted from 2023 to 2024 in South and Southwest China. WLWHAs from four hospitals were assigned using a WeChat-embedded randomization application to the control group (usual care) or the HOPES intervention. Data analysts remained blinded. Interventions were conducted virtually using Leave No One Behind (LNOB) platform for 3 months. The primary outcome, perceived stigma score, was assessed at baseline, immediately after the intervention and at 3 months post-intervention using 7 items from the HIV/AIDS Stigma Experience Questionnaire (HASEQ), with data analyzed through repeated measures analysis.RESULTS: Of 136 WLWHAs screened, we randomized 101 WLWHAs (50 HOPES; 51 controls). The HOPES group demonstrated a statistically significant reduction in perceived stigma scores immediately after the intervention (-3.86 points, 95 % CI: 5.34 to -2.38, P < .001) and at three months post-intervention (-5.83 points, 95 % CI: 7.20 to -4.47, P < .001) compared to the control group.CONCLUSION: The findings demonstrate HOPES' efficacy in reducing perceived stigma in WLWHA. However, the clinical significance of these changes needs further investigation. Future research should focus on defining meaningful patient-reported thresholds, assessing long-term impact, and optimizing delivery methods.Neighborhood physical environment satisfaction and aging attitudes in older Chinese adults: Differences by chronic condition status
AbstractXu, Z., Zhao, D., Mao, W., Wang, J., Yang, Z., Zheng, Y., & Wu, B. (2025). Aging and Health Research, 5(3). 10.1016/j.ahr.2025.100241AbstractBackground: With China's aging population and rising prevalence of chronic conditions, it is crucial to understand the factors shaping aging attitudes. Satisfaction with the neighborhood physical environment may play an important role, particularly among those with chronic health issues. To examine the association between neighborhood physical environment satisfaction and aging attitudes among older Chinese adults, and to explore differences across groups with and without chronic conditions. Methods: We analyzed data from 11,395 participants aged 60 and above from the 2020 China Longitudinal Aging Social Survey (CLASS). Stratified multiple regression analyses were conducted, comparing subgroups with chronic conditions (n = 8405, 73.76 %) and without chronic conditions. Results: Among participants with chronic conditions, higher satisfaction with road conditions (β = 0.382, P = 0.001), environmental sanitation (β = 0.286, P = 0.007), and road/street lighting (β = 0.288, P = 0.005) was significantly associated with more positive attitudes toward aging. No significant associations were found among participants without chronic conditions. Conclusions: Age-friendly neighborhood environments are important for promoting positive aging attitudes, particularly among older adults living with chronic conditions. These findings offer valuable insights for policymakers, researchers, and service providers aiming to support healthy aging in rapidly aging societies like China.Racial and ethnic disparities in the burden of non-obese type 2 diabetes using different anthropometric measurements
AbstractSui, J., Wu, B., Zheng, Y., Mo, Z., Dong, Q., Ðoàn, L. N., Yi, S. S., & Qi, X. (2025). Obesity Medicine, 53. 10.1016/j.obmed.2024.100573AbstractAIMS: Compare racial/ethnic disparities in the prevalence of non-obese type 2 diabetes (T2D) and the proportion of non-obese individuals among T2D patients.METHODS: This cross-sectional study used ICD-9/10 codes to ascertain T2D. Participants were classified as non-obese by BMI (<25 kg/m2 for normal weight; <23 kg/m2 for Asian Americans), waist circumference (<102 cm for males, <88 cm for females), and waist-to-hip ratio (<0.9 for males, <0.85 for females). The statistical analysis used marginal standardization of predicted probabilities from multivariable logistic regression to calculate the prevalence.KEY RESULTS: Among 276,736 participants (mean age 51.7, 61.2% female), non-obese T2D prevalence varied: 6.85% (BMI), 4.17% (waist circumference), 3.63% (waist-to-hip ratio). Asian participants had the highest prevalence of normal-weight T2D (2.70% vs. 1.92% in White, OR 1.44, 95% CI: 1.22-1.69) and non-obese T2D by waist circumference (8.04% vs. 3.36%, OR 2.61, 95% CI: 2.35-2.89). Black participants had the highest prevalence using waist-to-hip ratio (5.37% vs. 2.91%, OR 1.91, 95% CI: 1.80-2.03).CONCLUSION: Asian Americans showed higher non-obese T2D prevalence by BMI and waist circumference, while Black adults had higher prevalence by waist-to-hip ratio, suggesting different fat distribution patterns.School-Based Protective Factors for HIV Prevention in the United States: Secondary Analysis of the Youth Risk Behavior Survey 2015-2019
AbstractGarcia, D. R., Fletcher, J., Goldsamt, L., Bell, D. L., Zheng, Y., & Dunn Navarra, A. M. (2025). Journal of the Association of Nurses in AIDS Care, 36(1), 54-68. 10.1097/JNC.0000000000000501AbstractThis secondary analysis of the National Youth Risk Behavior Survey (years 2015-2019) examines associations between school-based protective factors (i.e., safe school environments and academic achievement) and HIV risk behaviors among sexually experienced adolescent gay and bisexual men (n = 644), a population with the highest prevalence of undiagnosed HIV infections. Demographics included Hispanics/Latinos (25%, n = 158), Other race/ethnicity (14%, n = 88), and non-Hispanic Blacks/African Americans (13%, n = 81). Adjusted models showed that protective factors reduced odds for early sexual debut, multiple sexual partners, sex under the influence of drugs/alcohol, and condomless sex, with an additive effect demonstrated when two protective factors were present. Hispanics/Latinos had greater odds of reporting multiple sexual partners and HIV testing, indicating opportunities for school-based HIV prevention and further research. Our findings provide support for school-based programs that aim to improve social and structural determinants of health and ultimately reduce adolescent HIV burdens.Association Between Video-Based Telemedicine Visits and Medication Adherence Among Patients With Heart Failure: Retrospective Cross-Sectional Study
AbstractZheng, Y., Adhikari, S., Li, X., Zhao, Y., Mukhopadhyay, A., Hamo, C. E., Stokes, T., & Blecker, S. (2024). JMIR Cardio, 8. 10.2196/56763AbstractBackground: Despite the exponential growth in telemedicine visits in clinical practice due to the COVID-19 pandemic, it remains unknown if telemedicine visits achieved similar adherence to prescribed medications as in-person office visits for patients with heart failure. Objective: Our study examined the association between telemedicine visits (vs in-person visits) and medication adherence in patients with heart failure. Methods: This was a retrospective cross-sectional study of adult patients with a diagnosis of heart failure or an ejection fraction of ≤40% using data between April 1 and October 1, 2020. This period was used because New York University approved telemedicine visits for both established and new patients by April 1, 2020. The time zero window was between April 1 and October 1, 2020, then each identified patient was monitored for up to 180 days. Medication adherence was measured by the mean proportion of days covered (PDC) within 180 days, and categorized as adherent if the PDC was ≥0.8. Patients were included in the telemedicine exposure group or in-person group if all encounters were video visits or in-person office visits, respectively. Poisson regression and logistic regression models were used for the analyses. Results: A total of 9521 individuals were included in this analysis (telemedicine visits only: n=830 in-person office visits only: n=8691). Overall, the mean age was 76.7 (SD 12.4) years. Most of the patients were White (n=6996, 73.5%), followed by Black (n=1060, 11.1%) and Asian (n=290, 3%). Over half of the patients were male (n=5383, 56.5%) and over half were married or living with partners (n=4914, 51.6%). Most patients’ health insurance was covered by Medicare (n=7163, 75.2%), followed by commercial insurance (n=1687, 17.7%) and Medicaid (n=639, 6.7%). Overall, the average PDC was 0.81 (SD 0.286) and 71.3% (6793/9521) of patients had a PDC≥0.8. There was no significant difference in mean PDC between the telemedicine and in-person office groups (mean 0.794, SD 0.294 vs mean 0.812, SD 0.285) with a rate ratio of 0.99 (95% CI 0.96-1.02; P=.09). Similarly, there was no significant difference in adherence rates between the telemedicine and in-person office groups (573/830, 69% vs 6220/8691, 71.6%), with an odds ratio of 0.94 (95% CI 0.81-1.11; P=.12). The conclusion remained the same after adjusting for covariates (eg, age, sex, race, marriage, language, and insurance). Conclusions: We found similar rates of medication adherence among patients with heart failure who were being seen via telemedicine or in-person visits. Our findings are important for clinical practice because we provide real-world evidence that telemedicine can be an approach for outpatient visits for patients with heart failure. As telemedicine is more convenient and avoids transportation issues, it may be an alternative way to maintain the same medication adherence as in-person visits for patients with heart failure.Association between visit frequency, continuity of care, and pharmacy fill adherence in heart failure patients
AbstractHamo, C. E., Mukhopadhyay, A., Li, X., Zheng, Y., Kronish, I. M., Chunara, R., Dodson, J., Adhikari, S., & Blecker, S. (2024). American Heart Journal, 273, 53-60. 10.1016/j.ahj.2024.04.003AbstractBackground: Despite advances in medical therapy for heart failure with reduced ejection fraction (HFrEF), major gaps in medication adherence to guideline-directed medical therapies (GDMT) remain. Greater continuity of care may impact medication adherence and reduced hospitalizations. Methods: We conducted a cross-sectional study of adults with a diagnosis of HF and EF ≤40% with ≥2 outpatient encounters between January 1, 2017 and January 10, 2021, prescribed ≥1 of the following GDMT: 1) Beta Blocker, 2) Angiotensin Converting Enzyme Inhibitor/Angiotensin Receptor Blocker/Angiotensin Receptor Neprilysin Inhibitor, 3) Mineralocorticoid Receptor Antagonist, 4) Sodium Glucose Cotransporter-2 Inhibitor. Continuity of care was calculated using the Bice-Boxerman Continuity of Care Index (COC) and the Usual Provider of Care (UPC) index, categorized by quantile. The primary outcome was adherence to GDMT, defined as average proportion of days covered ≥80% over 1 year. Secondary outcomes included all-cause and HF hospitalization at 1-year. We performed multivariable logistic regression analyses adjusted for demographics, insurance status, comorbidity index, number of visits and neighborhood SES index. Results: Overall, 3,971 individuals were included (mean age 72 years (SD 14), 71% male, 66% White race). In adjusted analyses, compared to individuals in the highest COC quartile, individuals in the third COC quartile had higher odds of GDMT adherence (OR 1.26, 95% CI 1.03-1.53, P = .024). UPC tertile was not associated with adherence (all P > .05). Compared to the highest quantiles, the lowest UPC and COC quantiles had higher odds of all-cause (UPC: OR 1.53, 95%CI 1.23-1.91; COC: OR 2.54, 95%CI 1.94-3.34) and HF (UPC: OR 1.81, 95%CI 1.23-2.67; COC: OR 1.77, 95%CI 1.09-2.95) hospitalizations. Conclusions: Continuity of care was not associated with GDMT adherence among patients with HFrEF but lower continuity of care was associated with increased all-cause and HF-hospitalizations.Age differences in the effects of multi-component periodontal treatments on oral and metabolic health among people with diabetes mellitus: A meta-epidemiological study
AbstractZhu, Z., Qi, X., Zheng, Y., Pei, Y., Wu, B., & Qi, X. (2023). Journal of Dentistry, 135, 104594. 10.1016/j.jdent.2023.104594AbstractOBJECTIVE: To explore the age differences in the effects of multi-component periodontal treatments on oral and metabolic indicators among individuals with periodontitis and diabetes.DATA: Trials reporting the effects of multi-component periodontal treatments on oral and metabolic indicators among participants aged 18 and above with periodontitis and diabetes were included.SOURCES: Six databases (PubMed/Medline, Embase, CINHAL, Web of Science, Cochrane Library, and ProQuest) were searched from database inception to August 2022.STUDY SELECTION: Two reviewers selected the included studies independently. We used bivariate and multivariate meta-regression models to examine the association between age and treatment effect size. The primary outcomes were changes in probing depth (PD), clinical attachment level (CAL), and hemoglobin A1c (HbA1c).RESULTS: A total of 18,067 articles were identified in the database search. Of these, 115 trials (119 articles) met inclusion criteria. The mean age of participants was 58 years old, ranging from 35 to 73 years. The pooled evidence demonstrated that multi-component periodontal treatment significantly reduced PD (g=0.929 [0.689-1.169], I2=94.1%), CAL (g=0.879 [0.669-1.089], I2=92.1%), and HbA1c (g=0.603 [0.443-0.763], I2=87.5%). A significant decreasing trend was observed in the effect size for PD (P for trend = 0.020) and CAL (P for trend = 0.028) as age increases. Results from multivariate meta-regression showed that mean age was associated with a smaller effect size for PD (β=-0.123 [0.041], P = 0.004) and CAL (β=-0.159 [0.055], P = 0.006). Compared to their younger counterparts, the effect size for HbA1c was smaller among participants aged 55 and older (β=-0.792 [0.322], P = 0.017).CONCLUSIONS: Multi-component periodontal treatments may be more effective in younger populations in terms of effects on PD, CAL, and HbA1c.CLINICAL SIGNIFICANCE: Our study highlights the importance of early intervention and tailored treatment approaches. Clinicians should take into account the patient's age when developing periodontal treatment plans and may need to employ more aggressive or personalized strategies for older adults to achieve optimal outcomes. -
-
Media
-
-
Active Projects